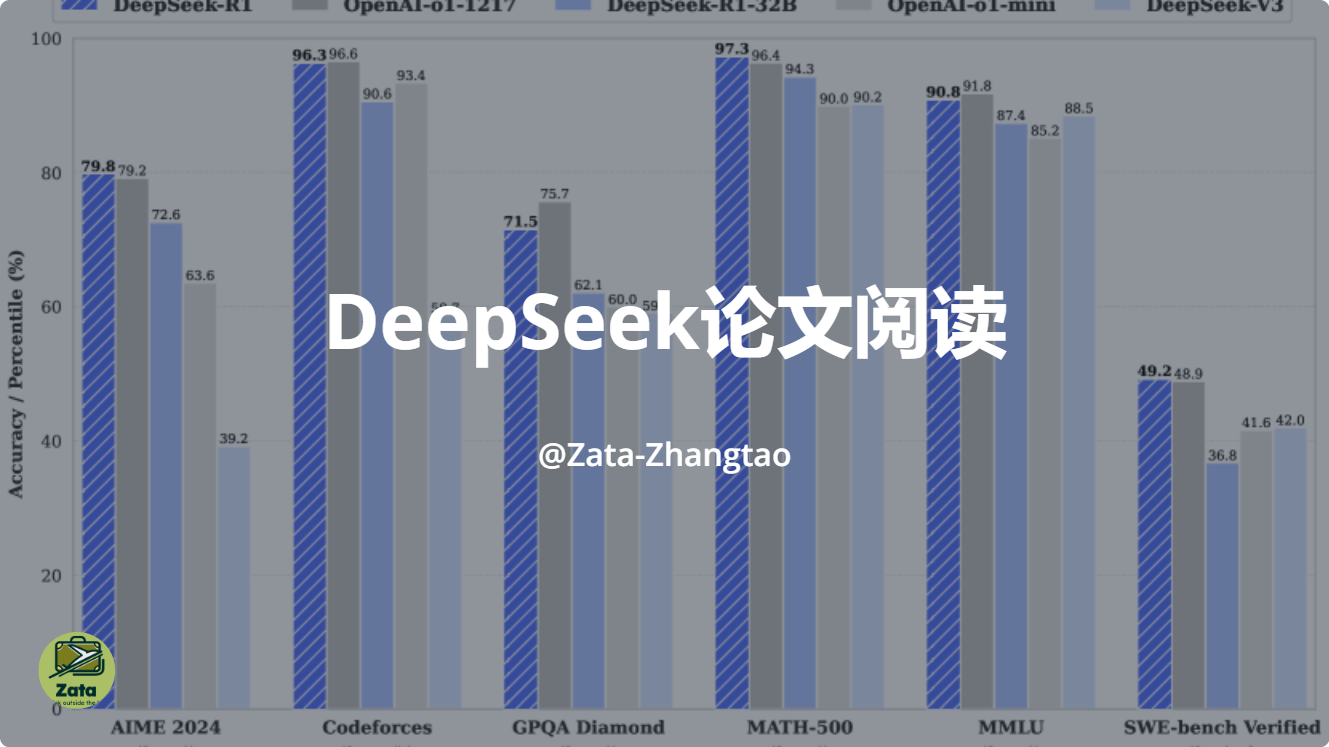
论文阅读:DeepSeek-R1: Incentivizing Reasoning Capability in LLMs via Reinforcement Learning
注意其中的这句话的意思是是基于R1的输出结果,微调的Qwen和Llama模型,也就是这个的基座模型是Qwen和Llama。从github上面可以看到它开源了如下模型其中有句话令人深思。
| 参考:
网上的解读
首先,Deepseek这么火了,让我们看看网上的解读
参考博客园
| 博客园
|https://zhuanlan.zhihu.com/p/20844750193
主要突破
研究者找到了一种新方法,让 AI 通过强化学习反复试错,逐渐学会像人一样多步推理问题
研究者先让一个基础模型(DeepSeek-V3-Base)直接进入强化学习,结果这个模型(称为 DeepSeek-R1-Zero)居然自己悟出了很多强大的解题技巧!比如,它学会了反思自己的答案、尝试不同思路等,这些都是人类优秀解题时会用的策略。
但是,仅靠自我摸索的 R1-Zero 也有明显的问题:它给出的答案有时很难读懂,甚至会中英混杂,或者回答偏离人们习惯的表达方式。为了解决这个问题,研究者对模型进行了两次额外的指导调整:第一次是喂给它一些"冷启动"例子。第二次是在强化学习之后,研究者收集了模型在训练中表现优秀的解题示例,再混合一些人工整理的题目,重新训练模型一次。经过这两轮调整,模型的表达流畅了,知识面也更广了。这时再让模型进行最后一轮强化学习,让它面对各种类型的问题训练,最终诞生的 DeepSeek-R1 模型,既有缜密的推理能力,又能用清晰自然的语言给出答案。
原文阅读
摘要
We introduce our first-generation reasoning models, DeepSeek-R1-Zero and DeepSeek-R1. DeepSeek-R1-Zero, a model trained via large-scale reinforcement learning (RL) without supervised fine-tuning (SFT) as a preliminary step, demonstrates remarkable reasoning capabilities. Through RL, DeepSeek-R1-Zero naturally emerges with numerous powerful and intriguing reasoning behaviors. However, it encounters challenges such as poor readability, and language mixing. To address these issues and further enhance reasoning performance, we introduce DeepSeek-R1, which incorporates multi-stage training and cold-start data before RL. DeepSeek-R1 achieves performance comparable to OpenAI-o1-1217 on reasoning tasks. To support the research community, we open-source DeepSeek-R1-Zero, DeepSeek-R1, and six dense models (1.5B, 7B, 8B, 14B, 32B, 70B) distilled from DeepSeek-R1 based on Qwen and Llama.
注意其中的
To support the research community, we open-source DeepSeek-R1-Zero, DeepSeek-R1, and six dense models (1.5B, 7B, 8B, 14B, 32B, 70B) distilled from DeepSeek-R1 based on Qwen and Llama.
这句话的意思是是基于R1的输出结果,微调的Qwen和Llama模型,也就是这个的基座模型是Qwen和Llama 。
https://github.com/deepseek-ai/DeepSeek-R1
从github上面可以看到它开源了如下模型
方法
这节是比较重要的了,文章介绍了具体的训练方法和发现
首先看概述
概述
Previous work has heavily relied on large amounts of supervised data to enhance model performance. In this study, we demonstrate that reasoning capabilities can be significantly improved through large-scale reinforcement learning (RL), even without using supervised fine-tuning (SFT) as a cold start. Furthermore, performance can be further enhanced with the inclusion of a small amount of cold-start data. In the following sections, we present: (1) DeepSeek-R1-Zero, which applies RL directly to the base model without any SFT data, and (2) DeepSeek-R1, which applies RL starting from a checkpoint fine-tuned with thousands of long Chain-of-Thought (CoT) examples. 3) Distill the reasoning capability from DeepSeek-R1 to small dense models.
其中有句话令人深思we demonstrate that reasoning capabilities can be significantly improved through large-scale reinforcement learning (RL), even without using supervised fine-tuning (SFT) as a cold start.
传统的思考模型是怎么样做的呢,这个主要借鉴openai的o1模型,在这个模型上面我们首先会构建预训练数据集,这个数据集和之前的对话模型不太一样,之前的对话模型的数据一般是【用户说:…,机器人说:…,用户说:…,机器人说:…,…】 也就是就两种数据,一个是机器人的话,一个是用户的话,但是思考模型的数据增加一个机器人思考的键值对,也就是现在变成了【用户说:…,机器人思考:…,机器人说:…,用户说:…,机器人思考:…,机器人说:…,…】也就是COT数据。
但是这个文章的这一段话,说:“我们不需要构建这样的数据集了”也就是不需要构建机器人思考这块内容,它就能自己去学会思考
强化学习算法
奖励模型
文章说到,设计了两种奖励模型,一种是准确率,也是大家通用的,和标准输出做对比。第二种就有意思了,Format rewards,前面我们也提到,R1模型不同于之前的o1模型相比,虽然输入数据格式上面不一样,但是输出要求是一样的,也是要求有一个思考内容存在,不过计算loss的时候,并没有标准思考内容做对比。
模型的训练
文章模型的训练可以简单看看,
然后有意思的一个点:
之前我看很多营销号说DeepSeek训练的时候突然出现了顿悟时刻,模型一下准确率变的很好,但是看了论文,实际上…
拒绝采样和监督微调部分
通过前面的方法,实际上是训练得到了R1-zero模型,即模型的思考过程是无监督的,但是现在需要得到人类可以看得懂的思考过程,因此需要对Zero模型进行微调,方法也是很常见的
其他内容
实验,讨论什么我们就不看了,可以看这段话
总有人喜欢做事后诸葛亮,我相信整个实现过程还是很难的,最终只是放出了成功的版本,而又有很多不成功的版本,这就是炼丹师。
更多推荐
所有评论(0)