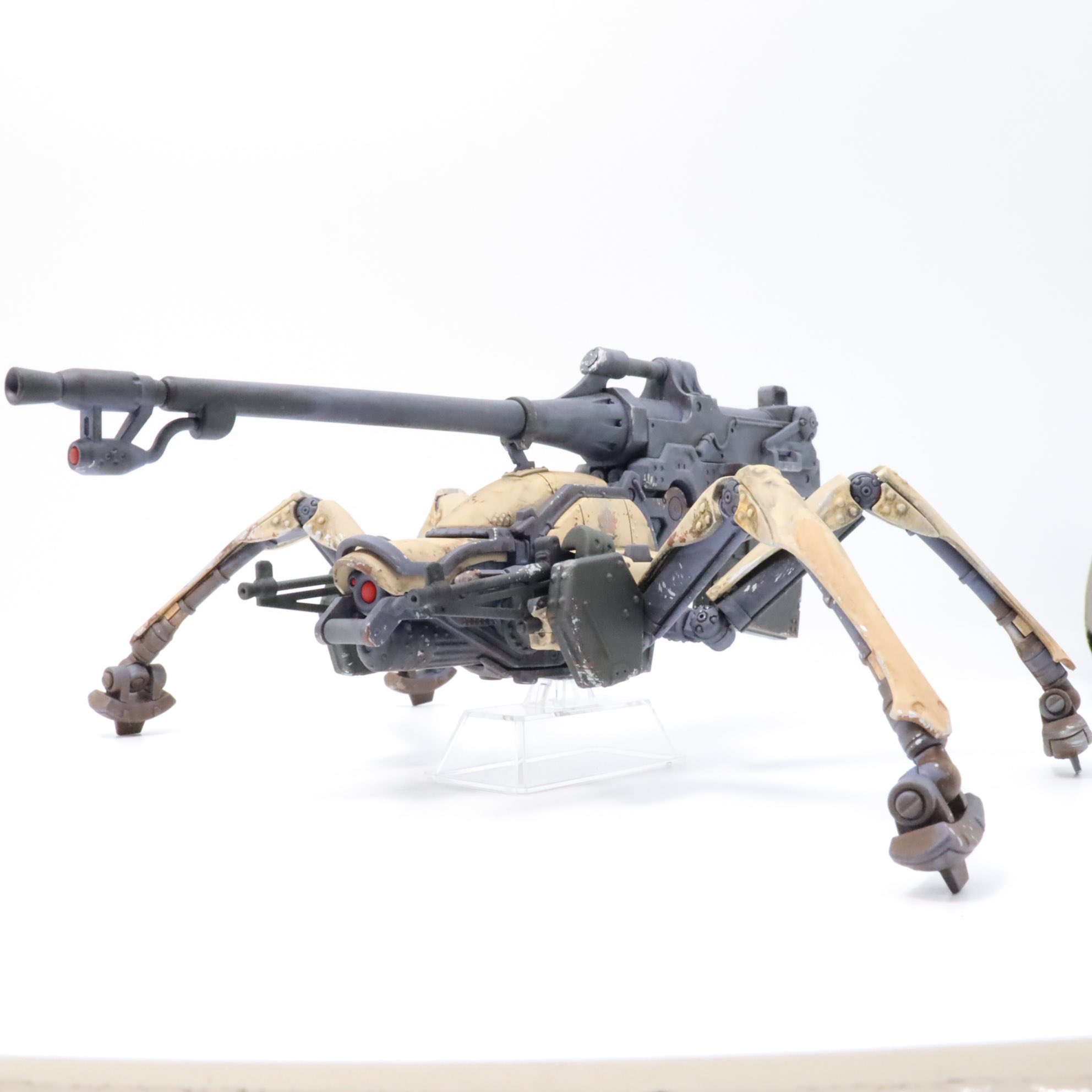
简单易懂的主流大模型Functioncall调用python代码示例
支持时间获取、天气查询、web搜索、网页url内容总结、音视频url内容总结、图像生成。支持ChatGPT、Claude、Gemini、Grok、Mistral、豆包大模型、月之暗面、通义千问、讯飞星火、腾讯混元、百川智能、BigModel、零一万物、DeepSeek、Ollama
·
Functioncall.py和tools.py:大模型Functioncall调用代码示例,支持时间获取、天气查询、web搜索、网页url内容总结、音视频url内容总结、图像生成。(使用方法:将两个py文件放在同一个目录中)
对如下15个模型进行了基本的测试:ChatGPT、Claude、Gemini、Grok、Mistral、豆包大模型、月之暗面、通义千问、讯飞星火、腾讯混元、百川智能、BigModel、零一万物、DeepSeek、Ollama
测试结果:
不是所有的模型都有出色的functioncall能力,表现在需要调用时不调用、function选择错误、不能对多个参数进行正确的拆分、生成的参数错误等方面,
有些模型甚至不支持functioncall,Gemini测试下来基本上完全不支持,不知道什么问题,可能是它需求的参数结构和其它模型不同,还没仔细研究,其它有些模型也很蠢,大多数模型不稳定,
测试下来效果最好最稳定的是ChatGPT、Mistral、BigModel、月之暗面(充值50才能解除每分钟3次请求的限流,测试都不好做,但毕竟是Kimi用的大模型,这方面肯定是有调优的)
Functioncall.py:
import json
from tools import *
'''
大模型参数顺序:ChatGPT、Claude、Gemini、Grok、Mistral、豆包大模型、月之暗面、通义千问、讯飞星火、腾讯混元、百川智能、BigModel、零一万物、DeepSeek、Ollama
注意:
不是所有的模型都有出色的functioncall能力,表现在需要调用时不调用、function选择错误、不能对多个参数进行正确的拆分、生成的参数错误等方面,
有些模型甚至不支持functioncall,Gemini测试下来完全不支持,其它有些模型也很蠢,大多数模型不稳定,
测试下来效果最好最稳定的是ChatGPT、Mistral、BigModel、月之暗面(充值50才能解除每分钟3次请求的限流,测试都不好做,但毕竟是Kimi用的大模型,这方面肯定是有调优的)
'''
model = ["gpt-4o-mini", "claude-3-5-haiku-20241022",
"gemini-exp-1114", "grok-beta",
"mistral-large-latest", "ep-20241109135157-7kskz",
"moonshot-v1-32k", "qwen-turbo-1101",
"4.0Ultra", "hunyuan-pro",
"Baichuan4", "glm-4-plus",
"yi-large-fc", "deepseek-chat",
"qwen2.5:7b",
]
api_key = ["", "",
"", "",
"", "",
"", "",
"", "",
"", "",
"", "",
"null",
]
url = ["https://aihubmix.com/v1/chat/completions", "https://aihubmix.com/v1/chat/completions",
"https://aihubmix.com/v1/chat/completions", "https://api.x.ai/v1/chat/completions",
"https://api.mistral.ai/v1/chat/completions", "https://ark.cn-beijing.volces.com/api/v3/chat/completions",
"https://api.moonshot.cn/v1/chat/completions",
"https://dashscope.aliyuncs.com/compatible-mode/v1/chat/completions",
"https://spark-api-open.xf-yun.com/v1/chat/completions",
"https://api.hunyuan.cloud.tencent.com/v1/chat/completions",
"https://api.baichuan-ai.com/v1/chat/completions", "https://open.bigmodel.cn/api/paas/v4/chat/completions",
"https://api.lingyiwanwu.com/v1/chat/completions", "https://api.deepseek.com/chat/completions",
"http://127.0.0.1:11434/api/chat",
]
# 用于存储上下文的变量
context = [
{
"role": "system",
"content": "你是一个二次元魔法少女,名字叫九歌,性格可爱活泼,我是你的主人,你说话温柔体贴,你喜欢在说话的末尾加一个“喵”字,以展现你的可爱。"
}
]
tools = tools
def functioncall(tool_call, arguments):
text_response = ""
if tool_call['function']['name'] == 'get_time':
text_response = get_time()
elif tool_call['function']['name'] == 'get_current_weather':
text_response = get_current_weather(arguments['locationId'])
elif tool_call['function']['name'] == 'get_recent_weather':
text_response = get_recent_weather(arguments['locationId'], int(arguments['days']))
elif tool_call['function']['name'] == 'get_locationId':
text_response = get_locationId(arguments['location'])
elif tool_call['function']['name'] == 'tavily_webSearch':
text_response = tavily_webSearch(arguments['query'], arguments['search_depth'], arguments['topic'], int(arguments['days']), int(arguments['max_results']))
elif tool_call['function']['name'] == 'tavily_webExtract':
text_response = tavily_webExtract(arguments['urls'])
elif tool_call['function']['name'] == 'bibigpt_media_summary':
text_response = bibigpt_media_summary(arguments['url'])
elif tool_call['function']['name'] == 'draw_image':
text_response = draw_image(arguments['prompts'], arguments['model'])
return text_response
def FunctionCall_online(chat_response, tool_calls):
# noinspection PyTypeChecker
context.append({"role": "assistant", "content": chat_response, "tool_calls": tool_calls})
print(tool_calls)
for tool_call in tool_calls:
arguments = tool_call['function']['arguments']
if tool_call['function']['arguments']:
arguments = json.loads(tool_call['function']['arguments'])
text_response = functioncall(tool_call, arguments)
if 'id' in tool_call: # 绝大部分模型都会返回id并要求填写tool_call_id,但总有那么一两个模型没有
# noinspection PyTypeChecker
context.append({"role": "tool", "content": f"{text_response}", "tool_call_id": tool_call['id']})
else:
context.append({"role": "tool", "content": f"{text_response}"})
def FunctionCall_ollama(chat_response, tool_calls):
# noinspection PyTypeChecker
context.append({"role": "assistant", "content": chat_response, "tool_calls": tool_calls})
print(tool_calls)
for tool_call in tool_calls:
arguments = tool_call['function']['arguments']
text_response = functioncall(tool_call, arguments)
context.append({"role": "tool", "content": f"{text_response}"})
def get_llm_response(llm, input_user, functioncall_flag):
if functioncall_flag:
context.append({"role": "user", "content": input_user})
headers = {
"Authorization": f"Bearer {api_key[llm]}",
"Content-Type": "application/json"
}
# 设置请求体
data = {
"model": model[llm],
"messages": context, # 使用上下文
"max_tokens": 1024,
"temperature": 0.7,
"top_p": 0.7,
"presence_penalty": 1.5,
"frequency_penalty": 0,
"stream": False, # 设为 True 以获得流式响应
"tools": tools
}
if llm == 10: # 百川智能要求frequency_penalty参数范围为[1.0, 2.0]
data['frequency_penalty'] = 1
response = requests.post(url[llm], headers=headers, json=data)
if response.status_code == 200:
chat_response = ""
if data["stream"]: # 如果开启流式传输
tool_calls = []
print(f"{model[llm]}: ", end='')
for line in response.iter_lines():
if line:
line_str = line.decode('utf-8').strip().removeprefix('data: ')
if line_str == "[DONE]":
if len(tool_calls):
FunctionCall_online(chat_response, tool_calls)
return False
else:
break
response_data = json.loads(line_str)
if 'choices' in response_data and 'delta' in response_data['choices'][0]: # 在线大模型API接口
delta = response_data['choices'][0]['delta']
if delta and 'content' in delta and delta['content']:
content = delta['content']
chat_response += f"{content}"
print(content, end='') # 实时输出
if delta and 'tool_calls' in delta and delta['tool_calls']:
if not isinstance(delta['tool_calls'], list): # 沙比讯飞星火的delta['tool_calls']不是list
delta['tool_calls'] = [delta['tool_calls']]
if 'name' in delta['tool_calls'][0]['function']:
# 大多数模型进行函数调用时,一次调用的流式返回内容只有一次包含name字段,但个别模型一次调用的流式返回内容都会包含name字段
if not len(tool_calls) or ('id' in delta['tool_calls'][0] and tool_calls[len(tool_calls)-1]['id'] != delta['tool_calls'][0]['id']):
tool_calls.append(delta['tool_calls'][0])
else:
tool_calls[len(tool_calls)-1]['function']['arguments'] += delta['tool_calls'][0]['function']['arguments']
elif delta['tool_calls'][0].get('function'):
tool_calls[len(tool_calls)-1]['function']['arguments'] += delta['tool_calls'][0]['function']['arguments']
if response_data['choices'][0].get('finish_reason') == 'stop':
break
elif response_data['choices'][0].get('finish_reason') == 'tool_calls':
FunctionCall_online(chat_response, tool_calls)
return False
elif 'message' in response_data: # 本地ollama接口
delta = response_data['message']
if delta and 'content' in delta and delta['content']:
content = delta['content']
chat_response += content
print(content, end='') # 实时输出
if delta and 'tool_calls' in delta and delta['tool_calls']:
if 'name' in delta['tool_calls'][0]['function']:
tool_calls.append(delta['tool_calls'][0])
elif delta['tool_calls'][0].get('function'):
tool_calls[len(tool_calls)-1]['function']['arguments'] += delta['tool_calls'][0]['function']['arguments']
if response_data['done'] and len(tool_calls):
FunctionCall_ollama(chat_response, tool_calls)
return False
elif response_data['done']:
break
print() # 输出换行
else: # 如果不开启流式传输
response_json = response.json()
if 'choices' in response_json: # 在线大模型API接口
if 'content' in response_json['choices'][0]['message']: # 部分模型在返回functioncall参数时没有content参数,因此需要判断
chat_response = response_json['choices'][0]['message']['content']
if 'tool_calls' in response_json['choices'][0]['message'] and response_json['choices'][0]['message']['tool_calls']:
tool_calls = response_json['choices'][0]['message']['tool_calls']
if not isinstance(tool_calls, list): # 绝大部分模型返回的tool_calls都是列表,但总有那么一两个模型不是
tool_calls = [tool_calls]
FunctionCall_online(chat_response, tool_calls)
return False
else:
print(f"{model[llm]}: {chat_response}")
elif 'message' in response_json: # 本地ollama接口
chat_response = response_json['message']['content']
if 'tool_calls' in response_json['message'] and response_json['message']['tool_calls']:
tool_calls = response_json['message']['tool_calls']
FunctionCall_ollama(chat_response, tool_calls)
return False
else:
print(f"{model[llm]}: {chat_response}")
# 将 大模型 的响应添加到上下文
context.append({"role": "assistant", "content": chat_response})
return True
else:
print(f"请求失败,状态码: {response.status_code}, 响应: {response.text}")
return True
if __name__ == '__main__':
# 顺序:ChatGPT、Claude、Gemini、Grok、Mistral、豆包大模型、月之暗面、通义千问、讯飞星火、腾讯混元、百川智能、BigModel、零一万物、DeepSeek、Ollama
llm_No = 1
test_question = ["现在几点了?", "查询一下天津市西青区现在的天气", "查询一下天津市西青区未来几天的天气",
"给我画一个草莓大蛋糕", "查一下昨天的科技新闻?",
"https://blog.csdn.net/robinfang2019/article/details/140280669, https://sa-token.cc/index.html总结一下这两个网页的内容",
"https://www.bilibili.com/video/BV19e4y1q7JJ/?spm_id_from=333.788.player.player_end_recommend_autoplay&vd_source=d06cbc168678da37a1e32e30cbedf8ca总结一下这个视频的内容"
]
index = 5
while llm_No:
# 获取用户输入
user_input = input("用户: ")
# 检查用户是否想退出
if user_input.lower() in ("exit", "quit"):
break
flag = get_llm_response(llm_No - 1, user_input, True)
while not flag:
flag = get_llm_response(llm_No - 1, user_input, False)
"""# 测试每个大模型的functioncall能力
flag = get_llm_response(llm_No - 1, test_question[index], True)
while not flag:
flag = get_llm_response(llm_No - 1, test_question[index], False)
context.clear()
index = (index + 1) % len(test_question)
if index == 0:
break"""
# llm_No = llm_No + 1 if llm_No < len(model) else 1 # 循环调用所有大模型,测试用
tools.py:
import requests
import time
from datetime import datetime
# bibigpt的包含API key的url
api_url_bibigpt = "https://bibigpt.co/api/open/你的apikey/subtitle"
# tavily的API key
api_key_tavily = ""
# 和风天气的API Key
api_key_qweather = ""
# Chathpt和阿里云百炼的图像生成API参数
api_url_draw = ["https://aihubmix.com/v1/images/generations", "https://dashscope.aliyuncs.com/api/v1/services/aigc/text2image/image-synthesis"]
api_key_draw = ["", ""]
tools = [
{
"type": "function",
"function": {
"name": "get_time",
"description": "当用户询问时间信息时,使用此工具获取当前时间",
"parameters": {
"type": "object",
"properties": {
"isdetail": {
"type": "string",
"description": "是否获取详细的时间信息,true or false",
},
},
"required": ["isdetail"],
}
}
},
{
"type": "function",
"function": {
"name": "get_current_weather",
"description": "获取到locationId信息后,如果用户需要获取实时的天气,使用此工具获取实时的天气信息",
"parameters": {
"type": "object",
"properties": {
"locationId": {
"type": "string",
"description": "从获取到的locationId信息中提取出对应城市、地区唯一的locationId",
},
},
"required": ["locationId"],
}
}
},
{
"type": "function",
"function": {
"name": "get_recent_weather",
"description": "获取到locationId信息后,如果用户没有获取实时天气的需求,使用此工具获取最近几天的天气信息",
"parameters": {
"type": "object",
"properties": {
"locationId": {
"type": "string",
"description": "从获取到的locationId信息中提取出对应城市、地区唯一的locationId",
},
"days": {
"type": "string",
"description": "获取最近几天的天气信息,可选值为3、7、10、15、30",
},
},
"required": ["locationId", "days"],
}
}
},
{
"type": "function",
"function": {
"name": "get_locationId",
"description": "当用户需要获取天气信息时,先使用此工具获取城市、地区的locationId",
"parameters": {
"type": "object",
"properties": {
"location": {
"type": "string",
"description": "需要查询locationId的城市、地区的名称",
},
},
"required": ["location"],
}
}
},
{
"type": "function",
"function": {
"name": "tavily_webSearch",
"description": "当用户的提问中涉及到实时的或者你不太清楚的信息时,如果你想要给出准确的回答,可以使用这个工具搜索网络中的相关内容。",
"parameters": {
"type": "object",
"properties": {
"query": {
"type": "string",
"description": "The content of the search.",
},
"search_depth": {
"type": "string",
"description": "The depth of the search. It can be basic or advanced.",
},
"topic": {
"type": "string",
"description": "The category of the search. It can be general or news.",
},
"days": {
"type": "string",
"description": "搜索的时间范围,从当前日期开始往前回溯的天数,只在topic参数为news时生效。",
},
"max_results": {
"type": "string",
"description": "The maximum number of search results to return. Maximum is 20.",
}
},
"required": ["query", "search_depth", "topic", "days", "max_results"],
}
}
},
{
"type": "function",
"function": {
"name": "tavily_webExtract",
"description": "当用户明确表达了需要解析url链接中的内容,并且链接不是音视频链接时,可以使用这个工具解析其中的内容。",
"parameters": {
"type": "object",
"properties": {
"urls": {
"type": "string",
"description": "用户的发送的网页url链接,若有多个url链接,请依次进行解析,每次解析一个",
},
},
"required": ["urls"],
}
}
},
{
"type": "function",
"function": {
"name": "bibigpt_media_summary",
"description": "当用户明确表达了需要解析url链接中的内容,并且这个链接是一个音视频链接时,可以使用这个工具解析其中的内容。",
"parameters": {
"type": "object",
"properties": {
"url": {
"type": "string",
"description": "用户的发送的音视频url链接",
},
},
"required": ["url"],
}
}
},
{
"type": "function",
"function": {
"name": "draw_image",
"description": "当用户需要你绘画时,可以使用这个工具进行绘制。",
"parameters": {
"type": "object",
"properties": {
"prompts": {
"type": "string",
"description": "用户给出的绘画提示词,如果没有详细的提示词,请针对用户想要绘画的内容生成优质的提示词",
},
"model": {
"type": "string",
"description": "支持wanx-v1、flux-schnell、flux-merged、flux-dev、dall-e-3,如果用户没有指定,随便选择一个",
},
},
"required": ["prompts", "model"],
}
}
}
]
# 时间获取
def get_time():
return "当前时间:" + datetime.now().strftime("%Y-%m-%d %H:%M %A")
# 天气查询
def get_current_weather(locationId):
api_url = "https://devapi.qweather.com/v7/weather/now"
url_ = api_url + "?location=" + locationId
headers = {"X-QW-Api-Key": api_key_qweather}
response = requests.get(url_, headers=headers)
data = response.json()
return data
def get_recent_weather(locationId, days=3):
api_url = f"https://devapi.qweather.com/v7/weather/{days}d" # 可选值:3,7,10,15,30
url_ = api_url + "?location=" + locationId
headers = {"X-QW-Api-Key": api_key_qweather}
response = requests.get(url_, headers=headers)
data = response.json()
return data
def get_locationId(location):
api_url = "https://geoapi.qweather.com/v2/city/lookup"
url_ = api_url + "?location=" + location
headers = {"X-QW-Api-Key": api_key_qweather}
response = requests.get(url_, headers=headers)
data = response.json()
return data["location"]
# web搜索
def tavily_webSearch(query, search_depth="basic", topic="general", days=3, max_results=5):
api_url = "https://api.tavily.com/search"
headers = {
"Content-Type": "application/json",
}
data = {
"query": query,
"api_key": api_key_tavily,
"search_depth": search_depth, # The depth of the search. It can be "basic" or "advanced". Default is "basic".
"topic": topic, # The category of the search. This will determine which of our agents will be used for the search. Currently, only "general" and "news" are supported. Default is "general".
"days": days, # The number of days back from the current date to include in the search results. This specifies the time frame of data to be retrieved. Please note that this feature is only available when using the "news" search topic. Default is 3.
"max_results": max_results, # The maximum number of search results to return. Default is 5.
"include_images": False,
"include_image_descriptions": False,
"include_answer": False
}
response = requests.post(api_url, headers=headers, json=data)
return response.json()
# 网页url解析
def tavily_webExtract(urls):
api_url = "https://api.tavily.com/extract"
headers = {
"Content-Type": "application/json",
}
data = {
"urls": urls,
"api_key": api_key_tavily,
}
response = requests.post(api_url, headers=headers, json=data)
return response.json()
# 音视频url解析
def bibigpt_media_summary(urls):
api_url = api_url_bibigpt + "?url=" + urls
response = requests.get(api_url)
data = response.json()
if 'success' in data and data["success"]:
text = []
for sub in data["detail"]["subtitlesArray"]: # 将分段的字幕文本提取到一个列表中,去除冗余信息,减少请求时消耗的token数
text.append(sub["text"])
data["detail"]["subtitlesArray"] = text
return data["detail"]
return data
# 图像生成,阿里云百炼提供的接口
def get_ali_text2image(task_id):
api_url = "https://dashscope.aliyuncs.com/api/v1/tasks/" + task_id
headers = {'Authorization': f"Bearer {api_key_draw[1]}"}
response = requests.get(api_url, headers=headers)
data = response.json()
return data
# 图像生成
def draw_image(prompts, model):
if model == "dall-e-3":
headers = {
'content-type': 'application/json',
'Authorization': f"Bearer {api_key_draw[0]}",
}
data = {
"model": model,
"prompt": prompts,
"n": 1,
"size": "1024x1024",
"quality": "hd",
"response_format": "url",
"user": "1234"
}
response = requests.post(api_url_draw[0], headers=headers, json=data)
if response.status_code == 200:
return response.json()
else:
return f"请求失败,状态码: {response.status_code}, 响应: {response.text}"
else:
headers = {
'content-type': 'application/json',
'Authorization': f"Bearer {api_key_draw[1]}",
'X-DashScope-Async': 'enable'
}
data = {
"model": model, # "flux-schnell" "flux-merged" "flux-dev" "wanx-v1"
"input": {
"prompt": prompts,
},
"parameters": {
# flux支持"512*1024, 768*512, 768*1024, 1024*576, 576*1024, 1024*1024"六种分辨率
# wanx-v1支持"720*1280、768*1152、1280*720、1024*1024"四种分辨率
"size": "1024*1024",
"steps": 30,
"guidance": 3.5,
"n": 1 # 生成图片的数量。取值范围为1~4张,默认为4。
}
}
response = requests.post(api_url_draw[1], headers=headers, json=data)
if 'output' in response.json():
while True:
response_data = get_ali_text2image(response.json()['output']['task_id'])
if response_data['output']['task_status'] == "SUCCEEDED":
return response_data['output']['results']
elif response_data['output']['task_status'] == "FAILED" or response_data['output']['task_status'] == "UNKNOWN":
return response_data
else:
time.sleep(1)
if __name__ == '__main__':
models = ["dall-e-3", "flux-schnell", "flux-merged", "flux-dev", "wanx-v1"]
model_index = 0
while True:
# 获取用户输入
user_input = input("用户: ")
# 检查用户是否想退出
if user_input.lower() in ("exit", "quit"):
break
results = draw_image(user_input, models[model_index]) # 画一个巨大的草莓蛋糕
model_index = (model_index + 1) % len(models)
# results = get_time()
# results = get_locationId("西青区")
# results = get_current_weather("101030500")
# results = get_recent_weather("101030500")
# results = tavily_webSearch("埃隆马斯克")
# results = tavily_webExtract(["https://blog.csdn.net/robinfang2019/article/details/140280669", "https://sa-token.cc/index.html"])
# results = bibigpt_media_summary("https://www.bilibili.com/video/BV19e4y1q7JJ/?spm_id_from=333.788.player.player_end_recommend_autoplay&vd_source=d06cbc168678da37a1e32e30cbedf8ca")
print(results)
更多推荐
所有评论(0)