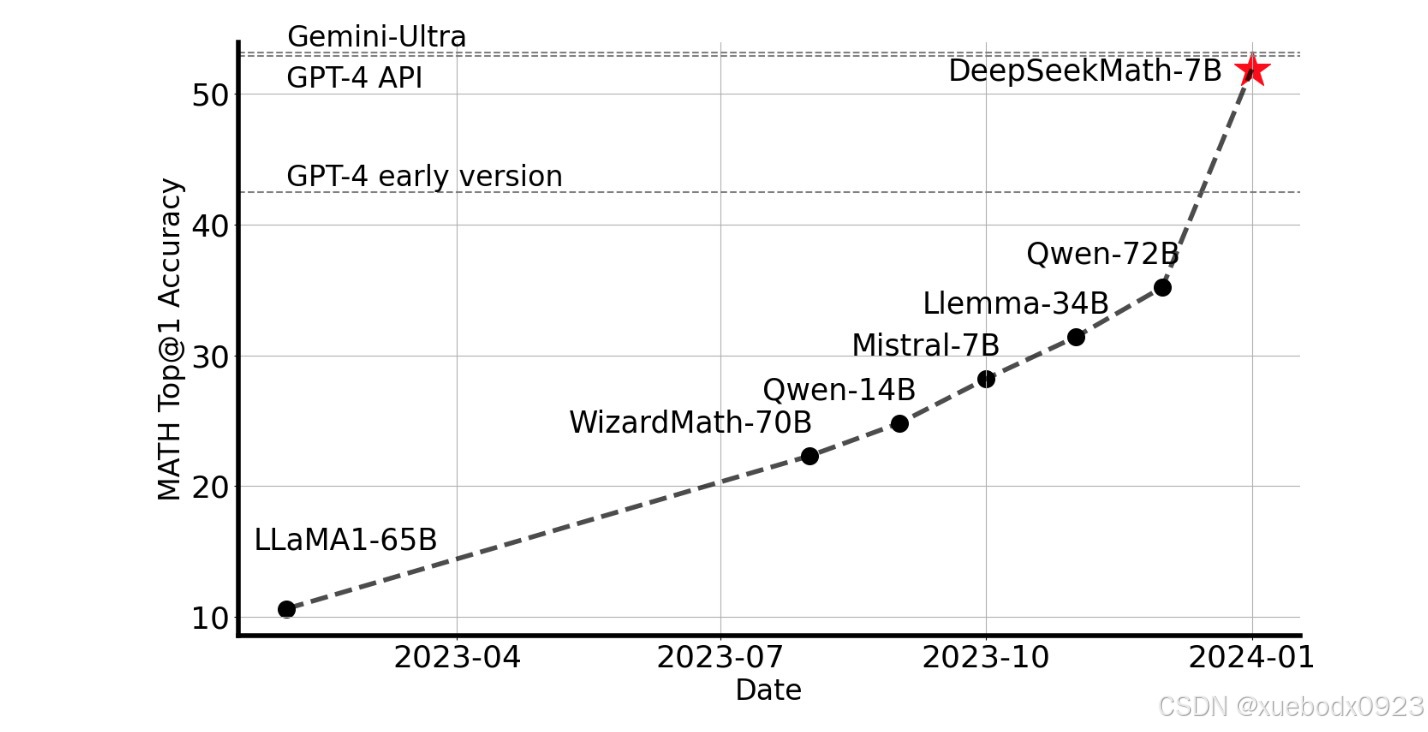
DeepSeek-Math解读
是DeepSeek推出的数学推理大模型,使用DeepSeek-Coder-v1.5-7B进行初始化,并继续对来自 Common Crawl 的数学相关tokens以及 500B 个tokens的自然语言和代码数据进行预训练。7B在不依赖外部工具包和投票技术的情况下,在竞赛级MATH基准上取得了令人印象深刻的51.7%的成绩,接近和GPT-4的性能水平。使用500B。
1. 摘要
DeepSeek-Math是DeepSeek推出的数学推理大模型,使用DeepSeek-Coder-v1.5-7B进行初始化,并继续对来自 Common Crawl 的数学相关tokens以及 500B 个tokens的自然语言和代码数据进行预训练。DeepSeek-Math-7B 在不依赖外部工具包和投票技术的情况下,在竞赛级 MATH 基准上取得了令人印象深刻的51.7%的成绩,接近 Gemini-Ultra 和 GPT-4 的性能水平。
2. DeepSeek-Math-7B-Base
使用500B个标记的自然语言和代码数据在DeepSeekCoder-Base-7B-v1.5模型上预训练,训练后的模型为DeepSeek-Math-7B-Base。
评估结果总结:
- 卓越的数学推理能力:在竞赛级 MATH 数据集上,DeepSeek-Math-7B-Base 通过少样本思路链提示,绝对性能超越现有开源基础模型 10% 以上,同时也超越了 Minerva 540B。
- 强大的工具使用能力:继续使用DeepSeekCoder-Base-7B-v1.5进行预训练,使得DeepSeek-Math-7B-Base能够通过编写程序更有效地解决和证明数学问题。
- 推理和编码性能:DeepSeek-Math-7B-Base 在推理和编码方面实现了与 DeepSeekCoder-Base-7B-v1.5 相当的性能。
3. DeepSeek-Math-7B-Instruct和DeepSeek-Math-7B-RL
DeepSeek-Math-7B-Instruct是在DeepSeek-Math-7B-Base模型的基础上训练出来的。DeepSeek-Math-7B-RL是在DeepSeek-Math-7B-Instruct模型的基础上训练出来的。使用的强化学习算法是组相对策略优化 (GRPO) 算法。
在4个英文和中文定量推理基准上评估了不使用和使用工具的数学性能。如表所示,DeepSeek-Math-7B-Instruct展示了强大的分步推理性能,而 DeepSeek-Math-7B-RL在工具的帮助下在数学上的准确率接近 60%,超越了所有现有的开源模型。
4. 数据收集
- 步骤 1:选择OpenWebMath(一组高质量的数学网络文本)作为训练 FastText 模型的初始种子语料库。
- 步骤 2:使用FastText模型从去重后的Common Crawl数据库中检索数学网页。
- 步骤3:通过统计分析确定潜在的数学相关领域。
- 步骤 4:手动注释这些与数学内容相关的已识别域中的 URL。
- 步骤 5:将链接到这些带注释 URL 的网页(但尚未收集)添加到种子语料库中。跳转到步骤 1,直到进行四次迭代。
经过四次迭代的数据收集,我们最终得到了35.5M个数学网页,总计120B tokens。
5. 本地部署模型
5.1 模型下载
https://huggingface.co/deepseek-ai/deepseek-math-7b-instruct/tree/main
5.2 Text Completion
import torch
from transformers import AutoTokenizer, AutoModelForCausalLM, GenerationConfig
model_name = "deepseek-ai/deepseek-math-7b-base"
tokenizer = AutoTokenizer.from_pretrained(model_name)
model = AutoModelForCausalLM.from_pretrained(model_name, torch_dtype=torch.bfloat16, device_map="auto")
model.generation_config = GenerationConfig.from_pretrained(model_name)
model.generation_config.pad_token_id = model.generation_config.eos_token_id
text = "The integral of x^2 from 0 to 2 is"
inputs = tokenizer(text, return_tensors="pt")
outputs = model.generate(**inputs.to(model.device), max_new_tokens=100)
result = tokenizer.decode(outputs[0], skip_special_tokens=True)
print(result)
5.3 Chat Completion
import torch
from transformers import AutoTokenizer, AutoModelForCausalLM, GenerationConfig
model_name = "deepseek-ai/deepseek-math-7b-instruct"
tokenizer = AutoTokenizer.from_pretrained(model_name)
model = AutoModelForCausalLM.from_pretrained(model_name, torch_dtype=torch.bfloat16, device_map="auto")
model.generation_config = GenerationConfig.from_pretrained(model_name)
model.generation_config.pad_token_id = model.generation_config.eos_token_id
messages = [
{"role": "user", "content": "what is the integral of x^2 from 0 to 2?\nPlease reason step by step, and put your final answer within \boxed{}."}
]
input_tensor = tokenizer.apply_chat_template(messages, add_generation_prompt=True, return_tensors="pt")
outputs = model.generate(input_tensor.to(model.device), max_new_tokens=100)
result = tokenizer.decode(outputs[0][input_tensor.shape[1]:], skip_special_tokens=True)
print(result)
更多推荐
所有评论(0)