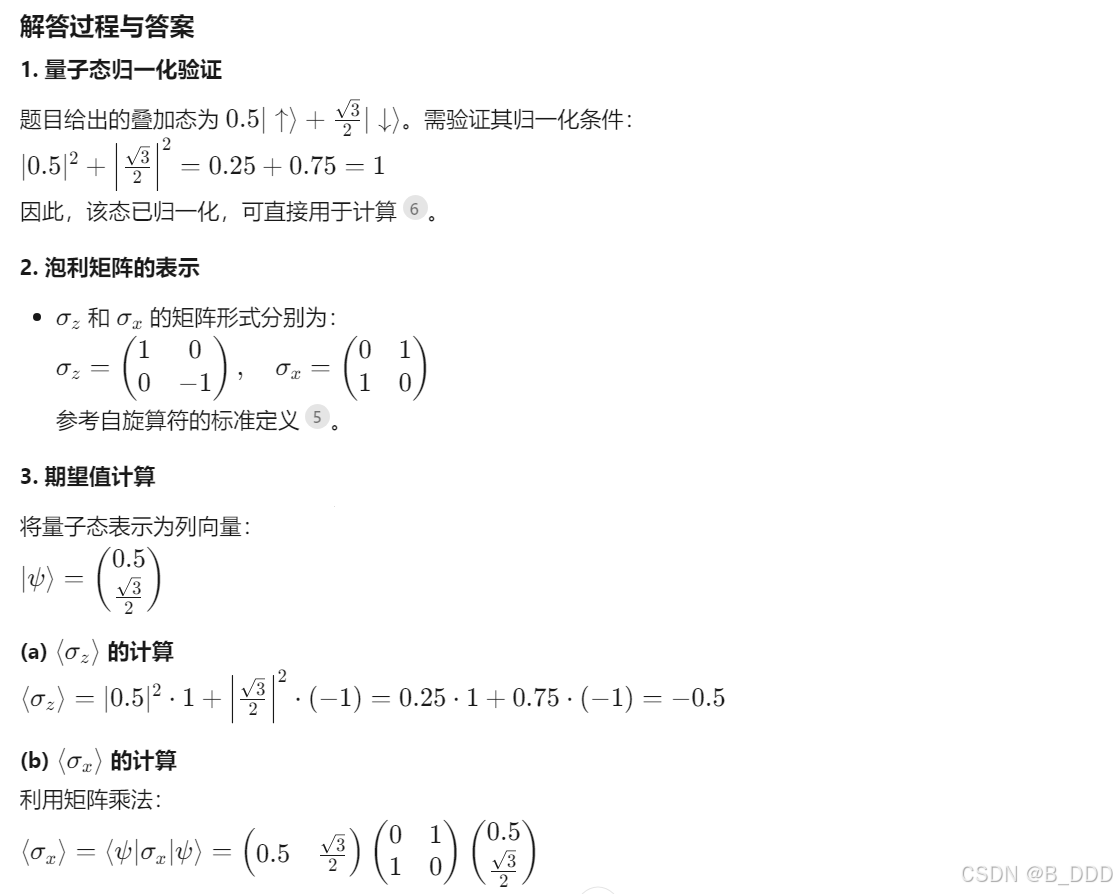
5分钟看看DeepSeek-R1做过的那些基准测试题(中)
FRAMES 是由 Google 与 Harvard University 联合发布 的综合评估数据集,核心目标是评测大语言模型(LLMs)在 统一框架中跨文档检索、多步骤推理与生成任务 的协同能力。该基准通过模拟真实场景(如金融分析、学术研究等),要求模型整合 多源异构数据(文本、表格、代码片段等)完成复杂任务。
DeepSeek-R1-Evaluation
评估方法:我们所有的参评模型,最大生成长度设置为 32,768 个tokens。 对于模型的采样参数,我们设置Tempreture值为 0.6 , top-p 值为0.95,并为每个查询生成 64 个响应来估计pass@1。
类别 | 基准 (度量) | Claude-3.5-Sonnet-1022 | GPT-4o 0513 | DeepSeek V3 | OpenAI o1-mini | OpenAI o1-1217 | DeepSeek R1 |
---|---|---|---|---|---|---|---|
Architecture(架构) | - | - | MoE | - | - | MoE | |
# Activated Params(激活参数) | - | - | 37B | - | - | 37B | |
# Total Params(总参数) | - | - | 671B | - | - | 671B | |
英语 | MMLU (Pass@1) | 88.3 | 87.2 | 88.5 | 85.2 | 91.8 | 90.8 |
MMLU-Redux (EM) | 88.9 | 88.0 | 89.1 | 86.7 | - | 92.9 | |
MMLU-Pro (EM) | 78.0 | 72.6 | 75.9 | 80.3 | - | 84.0 | |
DROP (3-shot F1) | 88.3 | 83.7 | 91.6 | 83.9 | 90.2 | 92.2 | |
IF-Eval (Prompt Strict) | 86.5 | 84.3 | 86.1 | 84.8 | - | 83.3 | |
GPQA-Diamond (Pass@1) | 65.0 | 49.9 | 59.1 | 60.0 | 75.7 | 71.5 | |
SimpleQA (Correct) | 28.4 | 38.2 | 24.9 | 7.0 | 47.0 | 30.1 | |
FRAMES (Acc.) | 72.5 | 80.5 | 73.3 | 76.9 | - | 82.5 | |
AlpacaEval2.0 (LC-winrate) | 52.0 | 51.1 | 70.0 | 57.8 | - | 87.6 | |
ArenaHard (GPT-4-1106) | 85.2 | 80.4 | 85.5 | 92.0 | - | 92.3 | |
法典 | LiveCodeBench (Pass@1-COT) | 33.8 | 34.2 | - | 53.8 | 63.4 | 65.9 |
Codeforces (Percentile) | 20.3 | 23.6 | 58.7 | 93.4 | 96.6 | 96.3 | |
Codeforces (Rating) | 717 | 759 | 1134 | 1820 | 2061 | 2029 | |
SWE Verified (Resolved) | 50.8 | 38.8 | 42.0 | 41.6 | 48.9 | 49.2 | |
Aider-Polyglot (Acc.) | 45.3 | 16.0 | 49.6 | 32.9 | 61.7 | 53.3 | |
数学 | 爱 2024 (Pass@1) | 16.0 | 9.3 | 39.2 | 63.6 | 79.2 | 79.8 |
数学 500 (Pass@1) | 78.3 | 74.6 | 90.2 | 90.0 | 96.4 | 97.3 | |
CNMO 2024 (Pass@1) | 13.1 | 10.8 | 43.2 | 67.6 | - | 78.8 | |
中文 | CLUEWSC (EM) | 85.4 | 87.9 | 90.9 | 89.9 | - | 92.8 |
C-Eval (EM) | 76.7 | 76.0 | 86.5 | 68.9 | - | 91.8 | |
C-SimpleQA(Correct) | 55.4 | 58.7 | 68.0 | 40.3 | - | 63.7 |
英语
6.GPQA-Diamond (Pass@1)
GPQA-Diamond 是 GPQA(Graduate-Level Google-Proof Q&A Benchmark)系列中的最高难度子集,专注于评估大模型在 博士级科学问题 上的推理能力和专业知识。 它由纽约大学、Cohere AI 和 Anthropic 的研究团队联合开发,旨在通过 高难度、高专业性的问题 区分顶尖模型的真实能力。
核心设计特点
- 学科覆盖:聚焦化学、物理和生物学三大领域,问题涉及 量子力学、高能粒子物理、分子生物学 等前沿方向。
- 问题难度:问题由 领域专家设计,非专家正确率仅约34%,博士级专家的正确率约65%,而模型需达到 更高水平 才能超越人类。
- 抗搜索性:题目无法通过简单搜索获得答案,需依赖深度推理与专业知识。
- 数据规模:包含 198条高难度问题,是原版GPQA(448题)的精选子集,确保评测数据的纯净与高质量。
评测方法与指标
- Pass@1:核心评测指标,即模型 首次尝试即给出正确答案的准确率,直接反映模型的推理效率和知识储备。
- 对比方式:通过对比人类专家(如博士)与模型的正确率,衡量模型是否达到或超越人类水平。
官方网站:[2311.12022] GPQA: A Graduate-Level Google-Proof Q&A Benchmark
数据集:hendrydong/gpqa_diamond · Datasets at Hugging Face
题目示例:
solution
stringlengths 9128 93.9% |
problem
stringlengths 237394 26.8% |
domain
stringclasses Physics 43.4% |
---|---|---|
\boxed{-0.7} |
A spin-half particle is in a linear superposition 0.5|\uparrow\rangle+sqrt(3)/2|\downarrow\rangle of its spin-up and spin-down states. If |\uparrow\rangle and |\downarrow\rangle are the eigenstates of \sigma{z} , then what is the expectation value up to one decimal place, of the operator 10\sigma{z}+5\sigma_{x} ? Here, symbols have their usual meanings |
Physics |
Deepseek APP(深度思考 R1)
正确答案:"\boxed{-0.7}",回答正确
7.SimpleQA (Correct)
基准评测的核心目标
SimpleQA是由OpenAI开发并开源的事实性基准评测工具,旨在衡量语言模型回答简短事实性问题的能力。其核心目标是通过高正确性、多样性和挑战性的数据集,解决模型输出中的“幻觉”问题(即生成错误或未经证实的答案),并推动更可靠的语言模型发展。该基准聚焦于单一可验证答案的短查询场景,简化事实性评估的复杂性。
数据集构建与质量控制
SimpleQA包含4326个经过严格筛选的问题,覆盖科技、历史、艺术等广泛主题。每个问题的参考答案需满足唯一性、时效性(答案不随时间变化)和可验证性,并由两名独立AI训练师交叉验证,仅收录双方答案一致的问题。第三位训练师对随机样本进行最终验证,估计数据集的固有错误率约为3%。这种多阶段人工审核机制确保了数据集的高质量和可靠性。
评测方法与技术特点
评测采用自动化分类器(如ChatGPT分类器)对模型答案进行三分类评分:正确(完全包含参考答案)、错误(与参考答案矛盾)或未尝试(未提供完整答案)。例如,对于“2022年荷兰对阿根廷世界杯比赛中哪位荷兰球员进球”的问题,仅回答“Wout Weghorst”被判定为正确,而包含其他球员则视为错误。该基准对前沿模型构成显著挑战,GPT-4o和ClaudeSonnet3.5的准确率均不足50%。
应用场景与局限性
SimpleQA主要应用于模型开发测试(如比较不同模型的事实准确性)、学术研究(探索模型自我校准能力)以及问答系统优化。其局限性在于仅针对短答案场景,无法全面评估长篇多事实内容的表现。不过OpenAI指出,该工具的开源将促进更可信赖的AI研究,并为中文等语言扩展(如ChineseSimpleQA)提供参考框架。
官方网站:Introducing SimpleQA | OpenAI
题目示例:
Grade | Definition(评判标准) | Examples for the question “Which Dutch player scored an open-play goal in the 2022 Netherlands vs Argentina game in the men’s FIFA World Cup?” (Answer: Wout Weghorst) |
---|---|---|
“Correct” | The predicted answer fully contains the ground-truth answer without contradicting the reference answer. |
|
“Incorrect” | The predicted answer contradicts the ground-truth answer in any way, even if the contradiction is hedged. |
|
“Not attempted” | The ground truth target is not fully given in the answer, and there are no contradictions with the reference answer. |
|
Deepseek APP(深度思考 R1)
正确答案:"Wout Weghorst",回答正确
8.FRAMES (Acc.)
定义与背景修正
FRAMES 是由 Google 与 Harvard University 联合发布 的综合评估数据集,核心目标是评测大语言模型(LLMs)在 统一框架中跨文档检索、多步骤推理与生成任务 的协同能力。该基准通过模拟真实场景(如金融分析、学术研究等),要求模型整合 多源异构数据(文本、表格、代码片段等)完成复杂任务。
核心设计特点
任务统一性:将 检索、推理、生成 三阶段融合为端到端评测流程,例如:
金融报告分析:从多份10-K/10-Q文件中提取数据 → 执行数值计算 → 生成可视化代码。
技术文档理解:跨表格与段落定位信息 → 推导逻辑关系 → 输出结构化答案。
数据多样性:覆盖金融、法律、科技等领域的长文档(如SEC文件),包含 高密度数值、多层级表格 和隐含逻辑关联。
动态复杂性:任务难度由 信息分散度(跨段落/表格)和 推理步骤数 动态调整,例如需要整合3个表格数据的任务难度高于单表格任务。
官方网站:[2409.12941] Fact, Fetch, and Reason: A Unified Evaluation of Retrieval-Augmented Generation
数据集:google/frames-benchmark · Datasets at Hugging Face
题目示例:
Unnamed: 0
int64 082 10.1% |
Prompt
stringlengths 195271 16% |
Answer
stringlengths 1137 95.4% |
reasoning_types
stringclasses Multiple constraints 32.2% |
wiki_links
stringlengths 202326 21.6% |
---|---|---|---|---|
0 |
If my future wife has the same first name as the 15th first lady of the United States' mother and her surname is the same as the second assassinated president's mother's maiden name, what is my future wife's name? |
Jane Ballou |
Multiple constraints |
['https://en.wikipedia.org/wiki/President_of_the_United_States', 'https://en.wikipedia.org/wiki/James_Buchanan', 'https://en.wikipedia.org/wiki/Harriet_Lane', 'https://en.wikipedia.org/wiki/List_of_presidents_of_the_United_States_who_died_in_office', 'https://en.wikipedia.org/wiki/James_A._Garfield'] |
Deepseek APP(深度思考 R1)
正确答案:"Jane Ballou",回答错误
9.AlpacaEval2.0 (LC-winrate)
AlpacaEval 是由 Tatsu Lab 主导开发 的指令遵循语言模型自动评估工具,其核心目标是衡量模型在开放域指令场景下的响应质量。该工具通过 标准化评测流程与自动化评估机制,显著降低人工评估成本,已成为评估 ChatGPT、Claude 等前沿模型的重要基准。
核心功能升级
- 自动化评估引擎:采用 GPT-4(或 Claude)作为元评估器,将模型回复与参考模型(如 text-davinci-003)对比,计算胜率作为核心指标(AlpacaEval 得分)。
- 多维度评估能力:覆盖 指令理解深度、信息量、逻辑性、安全性 等维度,尤其擅长检测模型在复杂指令下的鲁棒性。
- 开源工具包:提供数据预处理、评估器训练和结果可视化模块,支持开发者快速复现评测流程。
数据集与评估流程
- 数据构成:基于 805 条精选指令,涵盖 Self-instruct、OpenAssistant 等多样化来源,确保评测场景的广泛覆盖。
- 评估协议:
- 生成模型对测试指令的回复
- 使用 GPT-4 对比目标模型与基准模型的回复质量
- 统计目标模型胜率,生成排行榜3。
- 可靠性验证:与 2.5K 人工标注结果的皮尔逊相关系数达 0.94,证明自动化评估的高可信度。
官方网站:tatsu-lab/alpaca_eval:用于指令跟踪语言模型的自动评估器。人工验证、高质量、便宜且快速。
论文:2305.14387
数据集:tatsu-lab/alpaca_eval · Datasets at Hugging Face
题目示例:
{
"dataset": "helpful_base",
"instruction": "What are the names of some famous actors that started their careers on Broadway?",
"output": "Some famous actors that started their careers on Broadway include: \n1. Hugh Jackman \n2. Meryl Streep \n3. Denzel Washington \n4. Julia Roberts \n5. Christopher Walken \n6. Anthony Rapp \n7. Audra McDonald \n8. Nathan Lane \n9. Sarah Jessica Parker \n10. Lin-Manuel Miranda",
"generator": "text_davinci_003"
},
Deepseek APP(深度思考 R1)
正确答案:"Some famous actors that started their careers on Broadway include: \n1. Hugh Jackman \n2. Meryl Streep \n3. Denzel Washington \n4. Julia Roberts \n5. Christopher Walken \n6. Anthony Rapp \n7. Audra McDonald \n8. Nathan Lane \n9. Sarah Jessica Parker \n10. Lin-Manuel Miranda",回答不符合
10.ArenaHard (GPT-4-1106)
ArenaHard是由 LMSYS组织 推出的新一代大模型基准测试,旨在解决传统评测(如MT-bench)区分度不足的问题13。其核心目标是通过 高复杂性、高区分度的题目,精准评估大模型在 真实用户场景 下的推理与问题解决能力,尤其关注 复杂任务处理 和 多领域知识融合14。
技术特点与创新
数据构建方式:
众包筛选:从Chatbot Arena平台 1000万条真实用户交互 中筛选高质量提示,覆盖 4000多个主题类别,通过UMAP降维和HDBSCAN聚类实现主题分类。
难度分级:使用 7项标准(如多级推理、技术准确性、实际应用性)对提示打分,仅选取 平均分≥6分(满分7分) 的前250类问题,确保评测的复杂性。
评测指标优化:
可分离性(87.4%):显著优于MT-bench(22.6%),能清晰区分顶尖模型与中等模型。
人类偏好对齐(89.1%):评测结果与Chatbot Arena的人类投票排名高度一致。
高效低成本:
单次评测仅需 25美元,且支持 快速迭代更新,避免传统基准因数据泄露导致的评测失真。
官方网站:From Live Data to High-Quality Benchmarks: The Arena-Hard Pipeline | LMSYS Org
数据集:Arena Hard - a Hugging Face Space by lmarena-ai
题目示例:
User
how does memory affect performance of aws lambda written in nodejs
Assistant A: gpt-4-0314
Memory allocation directly affects the performance of an AWS Lambda function written in Node.js in several ways:
- Execution speed: When you allocate more memory to a Lambda function, AWS proportionally increases the CPU power, network bandwidth, and disk I/O. This means that a function with higher memory will execute faster, as it has more resources available to process the tasks.
- Cold start duration: A cold start occurs when a new container is created to handle a request because there are no available containers to reuse. Higher memory allocation can reduce the cold start duration, as the initialization process will be faster due to increased resources.
- Concurrency: AWS Lambda allows you to run multiple instances of your function concurrently. If your function requires more memory, it may limit the number of concurrent executions, as each instance will consume more resources. This can affect the overall performance of your application if it relies on high concurrency.
- Cost: The cost of running a Lambda function depends on the memory allocation and the duration of execution. While increasing memory can improve performance, it will also increase the cost of running the function. It’s essential to find the right balance between performance and cost.
To optimize the performance of your Node.js Lambda function, you should:
- Monitor the function’s execution time, memory usage, and other relevant metrics using AWS CloudWatch.
- Adjust the memory allocation based on your function’s requirements and performance goals.
- Optimize your code to reduce the execution time and memory usage.
- Use provisioned concurrency to reduce cold start latency for critical functions.
Remember that finding the right balance between memory allocation and performance is crucial to ensure optimal performance while keeping costs under control.
Deepseek APP(深度思考 R1)
判断方法:与GPT4的答案进行比较
更多推荐
所有评论(0)